The Biomedical Imaging and AI cluster is focused on increasing engagement and collaboration between our members. As part of this effort, we will be hosting monthly research exchange lunches.
All are welcome to attend, but the focus will be on student and trainee engagement. Participants will be asked to present their research or a research question in a lightning round format (3-5 minutes). Our recurring webinar series will encourage knowledge exchange among our cluster and support research collaboration.
If you are interested in presenting or attending, please sign up!
Details:
When: Last Wednesday of the Month, 12pm-1pm
Where: Zoom
June 23, 2021 | 12pm - 1pm (PST)
Digital Pathology
This month's theme of Digital Pathology features members from the Robotics Control Laboratory (RCL) and the AI in Medicine (AIM) group.
Speakers
Yanan Shao, PhD Candidate - supervised by Prof. Tim Salcudean
Title: Improving Prostate Cancer Classification in H&E Tissue Micro Arrays Using Ki67 and P63 Histopathology
Abstract
Histopathology of Hematoxylin and Eosin (H&E)-stained tissue obtained from biopsy is commonly used in prostate cancer (PCa) diagnosis. Automatic PCa classification of digitized H&E slides has been developed before, but no attempts have been made to classify PCa using additional tissue stains registered to H&E. In this paper, we demonstrate that using H&E, Ki67 and p63- stained (3-stain) tissue improves PCa classification relative to H&E alone. We also show that we can infer the PCa-relevant Ki67 and p63 information from the H&E slides alone and that we can use it to achieve H&E-based PCa classification that is comparable to the 3-stain classification. Reported improvements are both in classifying benign vs. malignant tissue, and low grade (Gleason group 2) vs. high grade (Gleason groups 3,4,5) cancer. Specifically, we conducted four classification tasks using 333 tissue samples extracted from 231 radical prostatectomy patients: regression tree-based classification using either (i) 3-stain features, with a benign vs malignant area under the curve (AUC=92.9%), or (ii) real H&E features and H&E features learned from Ki67 and p63 stains (AUC=92.4%), as well as deep learning classification using either (iii) real 3-stain tissue patches (AUC=94.3%) and (iv) real H&E patches and generated Ki67 and p63 patches (AUC=93.0%) using a deep convolutional generative adversarial network. Classification performance was assessed with Monte Carlo cross validation and quantified in terms of the Area Under the Curve, Brier score, sensitivity, and specificity. Our results are interpretable and indicate that the standard H&E classification could be improved by mimicking other stain types.
Jeffrey Boschman, MASc student - supervised by Prof. Ali Bashashati
Title: Improving Deep Learning Models for Clinical Epithelial Ovarian Carcinoma Whole Slide Pathology Image Classification using Color Normalization
Abstract
In order to provide the right treatment to a patient, a doctor needs to first diagnose the disease correctly. Although there are distinct subtypes of ovarian cancer, each with its own origin, features, aggressiveness, and treatment plans, many pathologists do not have the intensive training required to diagnose the subtype properly. This lack of specialists is part of the reason why ovarian cancer is the deadliest cancer of the female reproductive system in North America. Deep learning-based diagnostic models could supplement the pathologist laboratory, but the color variation of hematoxylin and eosin (H&E)-stained tissues has presented a challenge for applications of artificial intelligence (AI) in digital pathology. In this study, we systematically investigate eight color normalization algorithms for AI-based classification of H&E-stained histopathology slides, in the context of both using images from one center and from multiple centers. Our results show that color normalization does not consistently improve classification performance when both training and testing data are from a single center. However, using four multi-center datasets of two cancer types (ovarian and pleural) and objective functions, we show that color normalization can significantly improve the classification accuracy of images from external datasets (ovarian cancer: 0.25 AUC increase, p = 1.6 e-05, pleural cancer: 0.21 AUC increase, p = 1.4 e-10). Furthermore, we introduce a novel augmentation strategy by mixing color-normalized images using three easily accessible algorithms that consistently improves the diagnosis of test images from external centers, even when the individual normalization methods had varied results. We anticipate our study to be a starting point for reliable use of color normalization to improve AI-based, digital pathology empowered diagnosis of cancers sourced from multiple centers, including improving an ovarian cancer subtype diagnosis model to achieve the performance on par with specialist pathologists.
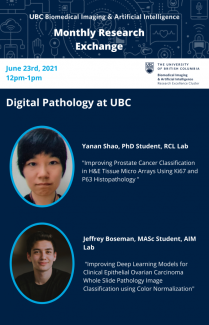